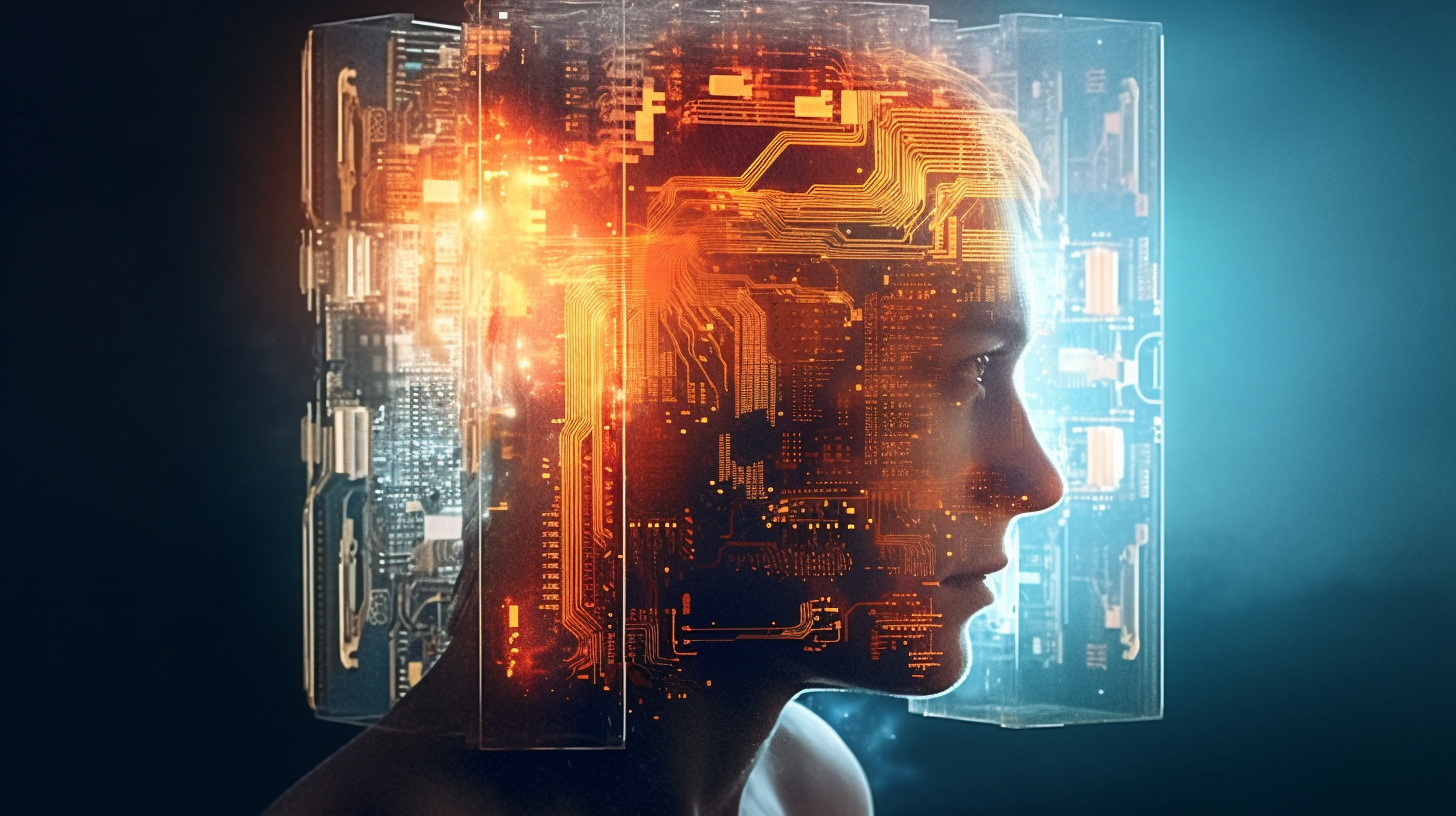
Available GPUs under 2000 Euros / Dollars
Based on the deep dive internet research performed by Trimtask agents, the following GPUs are available under the budget of 2000 Euros:
• Radeon RX 6600 (non-XT)
• GTX 1650 (or 1650S)
• Intel Arc A750
• Used AMD Radeon Cards: RX 5500 XT, RX 570/580/590
• Used Nvidia GeForce Cards: GTX 1660, GTX 1660 Super/Ti, RTX 2060
• GeForce RTX 4090
• Radeon RX 7900 XTX
• RTX 4070 Ti
• RTX 4080
• RTX 3060
• RX 6950 XT
• RX 6900 XT
• RX 6800 XT
• RX 6800
• RX 6650 XT
• Arc A770 8GB
• Arc A380
*Please note that the prices of some GPUs are not specified, and the used market prices may vary. The prices mentioned are current online prices alongside the official launch MSRPs.
Performance in Terms of Benchmarks and Specifications
The performance of these GPUs can be found in the Tom’s Hardware GPU benchmarks hierarchy. The website provides benchmark results for various GPUs, including the ones listed above. The results are based on 1080p ultra settings for the main suite and 1080p medium settings for the DXR suite. Factors such as price, power consumption, overall efficiency, and features are not factored into the rankings.
Technology Areas Comparisons
The GPUs listed above excel in different technology areas, such as gaming, content creation, and AI applications. Nvidia’s GPUs, for example, are known for their strong performance in AI and deep learning tasks, while AMD’s GPUs are often praised for their price-to-performance ratio in gaming. Intel’s Arc GPUs are relatively new to the market and are currently more focused on competing with previous-generation midrange offerings.
Support for Stable Diffusion and Large Language Models
Stable Diffusion can be run on many consumer-grade GPUs, with the most powerful Ampere GPU (A100) being only 33% faster than the 3080 when outputting a single image. The A100 80GB has the highest throughput due to its larger maximum batch size. Half-precision reduces the time by about 40% for Ampere GPUs and by 52% for the previous generation RTX8000 GPU. Memory usage is consistent across all tested GPUs, with single-precision inference with batch size one taking about 7.7 GB GPU memory and half-precision inference with batch size one taking about 4.5 GB GPU memory. Nvidia’s GPUs perform the best for Stable Diffusion, with the RTX 40-series cards being the fastest choice, followed by the 7900 cards, and then the RTX 30-series GPUs.
The RX 6000-series underperforms, and Intel’s Arc GPUs currently deliver very disappointing results.
Techniques to Deploy Large Language Models on CPUs (and high-level comparisons with GPU deployment)
Deploying large language models (LLMs) on CPUs using GPUs is a challenging task that requires advanced techniques such as model parallelism and quantization to achieve latency and throughput requirements.
The decision to choose the right type of hardware for deep learning tasks should be dependent on the task at hand and based on factors such as throughput requirements and cost.
GPUs are widely accepted for deep learning training due to their significant speed when compared to CPUs. However, for tasks like inference, which are not as resource-heavy as training, CPUs are usually believed to be sufficient and more attractive due to their cost savings. But when inference speed is a bottleneck, using GPUs provides considerable gains both from financial and time perspectives.
Several tests conducted in this area have concluded that for deep learning inference tasks which use models with a high number of parameters, GPU-based deployments benefit from the lack of resource contention and provide significantly higher throughput values compared to a CPU cluster of similar cost.
It is important to note that, for standard machine learning models where the number of parameters is not as high as deep learning models, CPUs should still be considered more effective and cost-efficient. Also, there exist methods to optimize CPU performance and similar optimizations can be achieved on GPU’s too.